Unlocking the Potential of Medical Datasets for Machine Learning
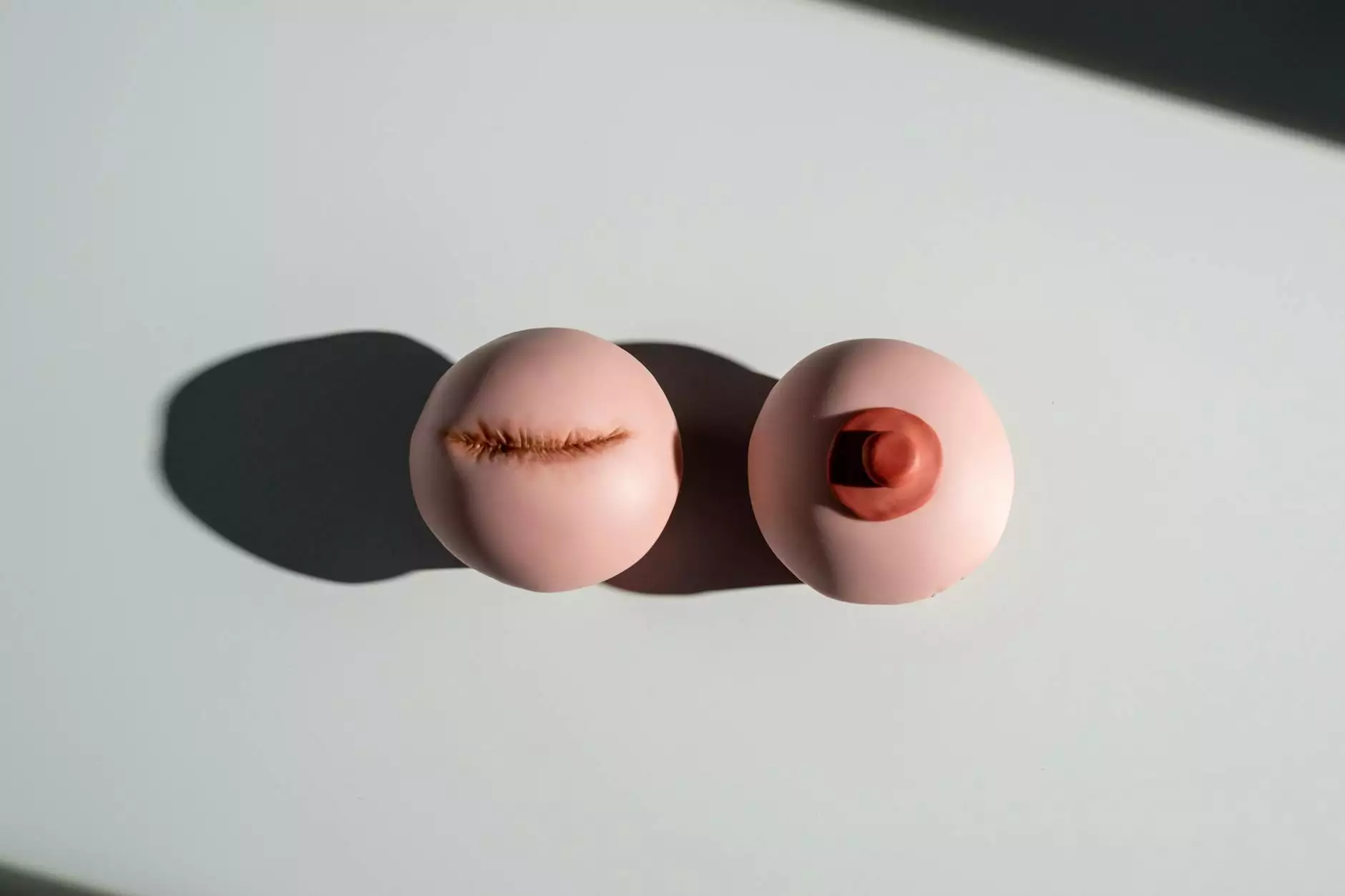
In today’s rapidly evolving technological landscape, the intersection of medicine and machine learning is creating opportunities that were once merely speculative. The availability of diverse and comprehensive medical datasets for machine learning represents a seismic shift in how we approach healthcare challenges. This article delves deeply into the significance of these datasets, their applications, and the immense benefits they offer to various stakeholders in healthcare.
The Importance of Medical Datasets
Medical datasets serve as the foundation for machine learning algorithms that can analyze complex healthcare data. Understanding the potential of these datasets is crucial for:
- Improving Patient Outcomes: Machine learning can enhance diagnosis and treatment decision-making.
- Streamlining Healthcare Operations: Automated processes increase the efficiency and effectiveness of healthcare delivery.
- Advancing Medical Research: Rich datasets enable researchers to expedite discoveries in treatments and interventions.
What Constitutes a Quality Medical Dataset?
A quality medical dataset for machine learning should have the following characteristics:
- Comprehensiveness: It should cover a wide range of medical conditions and demographics.
- Accuracy: Data must be precise and verified to ensure reliable outcomes.
- Accessibility: Datasets should be easy to access while maintaining strict ethical standards.
- Timeliness: Continuous updates are essential for the relevance of the dataset in the fast-evolving medical landscape.
Types of Medical Datasets Utilized in Machine Learning
Machine learning in healthcare utilizes various types of datasets, each serving specific functions. Here are several notable examples:
1. Electronic Health Records (EHR)
EHRs contain comprehensive patient information, including demographics, medical history, medication lists, and treatment plans. Mining data from EHRs can help predict health outcomes and identify trends in disease occurrence.
2. Genomic Data
Genomic datasets are pivotal for personalized medicine. They allow machine learning models to identify genetic markers associated with specific conditions, paving the way for tailored therapies.
3. Medical Imaging
Datasets consisting of X-rays, MRIs, and CT scans enable deep learning models to improve diagnostic accuracy and assist radiologists in detecting abnormalities that may be missed by the naked eye.
4. Clinical Trials Data
This data, collected from clinical trials, provides insights into drug efficacy and safety, and helps machine learning models predict patient responses to various treatments.
Applications of Machine Learning with Medical Datasets
Machine learning applications powered by robust medical datasets are myriad. Below are some of the most impactful:
1. Predictive Analytics
Healthcare providers utilize predictive analytics to foresee patient deterioration and proactively intervene. By feeding historical patient data into machine learning models, providers can forecast outcomes and optimize patient care.
2. Image Recognition in Radiology
Machine learning algorithms are actively employed to analyze medical images. These algorithms can identify and categorize abnormalities with exceptional precision, significantly reducing the workload of radiologists.
3. Personalized Treatment Plans
Integrating machine learning with genomic datasets allows clinicians to determine customized treatment plans based on a patient’s genetic makeup, enhancing treatment effectiveness and reducing adverse effects.
4. Drug Discovery
Machine learning accelerates the drug discovery process by analyzing large datasets to identify potential candidates for new medications, significantly shortening the timeline for bringing new treatments to market.
Challenges and Ethical Considerations
Despite the promising opportunities presented by medical datasets for machine learning, challenges persist:
1. Data Privacy Concerns
With healthcare data being highly sensitive, ensuring privacy and compliance with regulations such as HIPAA is paramount. Organizations must implement robust security measures to protect patient information.
2. Data Imbalance
A common challenge in machine learning is data imbalance, where certain medical conditions are underrepresented. This can lead to biased models that may perform poorly on less common conditions.
3. Ensuring Data Quality
Quality control is essential, as inaccuracies in data can lead to erroneous conclusions and detrimental patient outcomes. Continuous monitoring and updating of datasets are vital for maintaining high standards.
Future Trends in Medical Datasets and Machine Learning
The future of medical datasets for machine learning is bright and full of promise. Here are some anticipated trends:
1. Integration of Real-World Data
As the healthcare landscape evolves, integrating real-world evidence and data into machine learning models will enhance their accuracy and applicability.
2. Growth of Open-Source Datasets
More organizations are sharing anonymized medical datasets to help advance research and innovation, contributing to collaborative efforts in improving healthcare solutions.
3. Advances in Natural Language Processing (NLP)
NLP technologies will increasingly be applied to extract valuable insights from unstructured data, such as clinical notes, enhancing the value of EHRs and other text data.
4. Enhanced Interoperability
Improving the interoperability of systems will allow for seamless data sharing across municipal, regional, and national healthcare efforts, maximizing the utility of medical datasets.
Conclusion
In conclusion, the utilization of medical datasets for machine learning is not simply an emerging trend; it is a revolutionary development in the healthcare landscape. The synthesis of advanced technologies and comprehensive data has the potential to unlock valuable insights, improve patient care, and drive innovation in medical research.
As we continue to navigate the complexities and challenges presented by healthcare data, the focus must remain on creating robust, ethical, and accessible datasets that empower healthcare professionals and researchers alike. The future of medicine depends on how effectively we can harness data and technology to enhance patient outcomes and streamline healthcare services.
By investing in high-quality medical datasets and leveraging the power of machine learning, we can lay the groundwork for a healthier tomorrow. The journey has just begun, and the potential is limitless.