Labeling Tools for Machine Learning: The Essential Guide
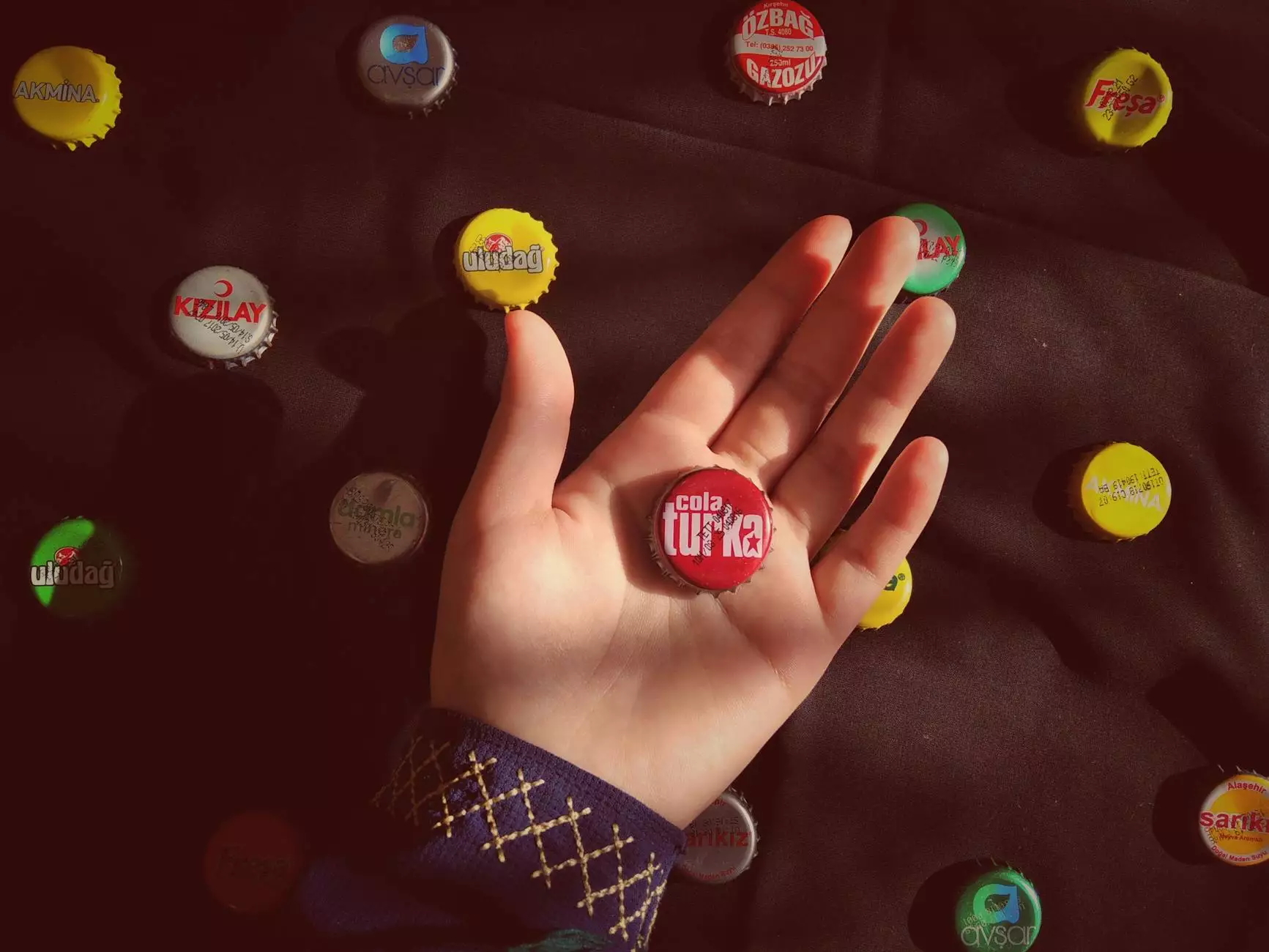
Labeling tools for machine learning are pivotal in transforming raw data into valuable information that can enhance artificial intelligence models. In an era where data is abundant, the ability to accurately annotate datasets is vital for the successful training of machine learning algorithms. This article delves deep into the realm of data annotation tools and platforms, particularly highlighting the offerings from KeyLabs.ai.
Understanding the Importance of Data Annotation
The foundation of machine learning lies in its ability to learn from data. However, not all data is actionable. This is where data annotation comes into play. Properly labeled datasets allow machine learning models to understand and learn patterns effectively. Here’s why data annotation is crucial:
- Accuracy in Predictions: Well-labeled data leads to more accurate predictions. Machine learning algorithms thrive on clear and precise information.
- Quality Model Training: High-quality annotations ensure that the models trained on this data deliver reliable results.
- Application Versatility: Different applications (from image recognition to natural language processing) require unique labeling approaches.
Types of Labeling Tools for Machine Learning
When it comes to machine learning, there are various types of labeling tools tailored for specific data types:
1. Image Annotation Tools
These tools facilitate the labeling of images for tasks such as object detection, image segmentation, and classification. Popular annotations include bounding boxes, polygons, and landmarks.
2. Text Annotation Tools
Text data requires unique labeling techniques to extract useful information. This includes tasks like named entity recognition, sentiment analysis, and categorization.
3. Video Annotation Tools
Video content can be annotated for action recognition, object tracking, and scene analysis. These tools allow for frame-by-frame labeling to ensure comprehensive data input.
Features of Effective Labeling Tools
Choosing the right labeling tools for machine learning is critical. Here are some key features that effective tools should possess:
- User-Friendly Interface: An intuitive design makes it easier for users to annotate data without extensive training.
- Collaboration Capabilities: Teams can work concurrently on a project, ensuring efficient use of resources.
- Integration with Machine Learning Frameworks: Seamless integration with popular ML frameworks like TensorFlow and PyTorch enhances workflow.
- Quality Control Mechanisms: Built-in quality checks ensure that the data annotated meets the required standards.
The Data Annotation Process
The process of data annotation can be broken down into several steps:
1. Defining Objectives
Before annotating data, it’s crucial to understand the specific goals of your machine learning project. What outcomes do you expect, and how will the data be used? Clear objectives guide the annotation process.
2. Selecting the Right Dataset
A dataset must be relevant and representative of the real-world scenarios it aims to address. Quality over quantity is key here.
3. Annotation Guidelines
Developing clear guidelines for annotators helps maintain consistency. These guidelines should detail how to label data accurately based on defined objectives.
4. Annotation Execution
Using labeling tools for machine learning, annotators begin labeling the data. Regular reviews and revisions may be necessary during this phase.
5. Quality Assurance and Final Review
Once annotation is complete, implementing quality control measures ensures that the labeled data meets the required accuracy before it's utilized for model training.
KeyLabs.ai: Your Partner in Data Annotation
At KeyLabs.ai, we provide state-of-the-art data annotation tools and platforms designed to meet the diverse needs of machine learning projects. Here’s why you should consider our solutions:
Comprehensive Annotation Solutions
Our tools support various types of data, including images, text, and video. We understand the differences in annotating each type and provide customized workflows.
Advanced Technology
Leveraging cutting-edge technology, our platforms are built with robust features that enhance productivity and accuracy. Our tools integrate seamlessly with existing workflows, making the annotation process fluid.
Expert Support
We believe in empowering our users. Our expert team is always available to assist with onboarding, support, and best practices in data annotation.
Challenges in Data Annotation
While progressing in the field of machine learning and data annotation, various challenges may arise:
- Scalability: As the volume of data increases, maintaining quality and speed can become difficult.
- Annotation Consistency: Different annotators may interpret guidelines differently, risking inconsistencies in the data quality.
- Budget Constraints: High-quality manual annotation can be resource-intensive and costly.
Future Trends in Data Annotation
As machine learning continues to evolve, so does the landscape of data annotation. Here are some exciting trends to watch:
1. Automation of Annotation
With advancements in AI, more tools are incorporating automated annotation features, reducing the need for manual labor, and increasing efficiency.
2. Crowdsourcing Annotations
Crowdsourcing platforms are emerging as a viable solution to manage large annotation tasks quickly and affordably, tapping into a global pool of annotators.
3. Integration with AI and Machine Learning
Tools are increasingly integrating AI solutions to assist and enhance human annotators, thus streamlining the annotation process while maintaining quality.
Conclusion
In conclusion, labeling tools for machine learning are essential assets in the development and success of AI models. Understanding the importance of accurate data annotation not only enhances the quality of machine learning models but ensures they derive intelligent insights from the data. With reliable partners like KeyLabs.ai, businesses can navigate the complexities of data annotation, empowering their AI initiatives and driving innovation.
Whether you're starting a new project or looking to optimize your existing dataset, investing in effective labeling tools is a strategic move toward realizing your machine learning ambitions.